Zobrazení pro čtení
Noctuary Review – A Stunning Visual Novel RPG Worth Discovering
Arcade shooter Nova Drift is a Petri dish in which to spawn the daftest, deadliest spaceship
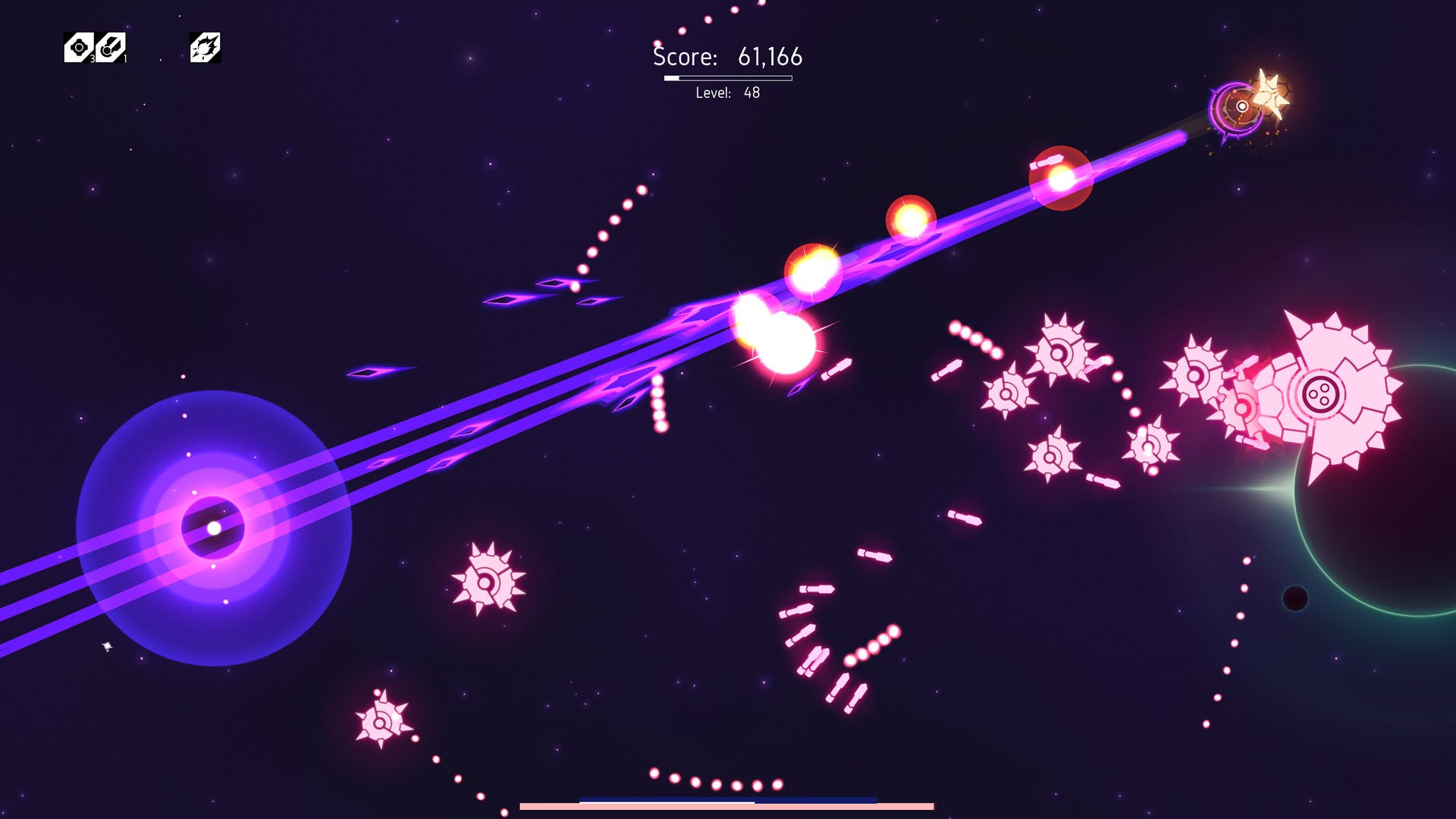
I'm no shoot 'em up nutter - or "shmutter", as I understand they prefer to be called - but some of the first games I remember playing are shmups. Games like Maelstrom, Ambrosia's Macintosh clone of Asteroids, and the proto-shmup Crystal Quest from Patrick Buckland, who would go on to make Carmageddon. Little did I know that the humble premise of a small 2D spacecraft shooting baddies on a wrap-around screen would reach the glittering heights of Nova Drift. Had you shown me this game back in 1995, I dare say I'd have shmupped myself.
Arcade shooter Nova Drift is a Petri dish in which to spawn the daftest, deadliest spaceship
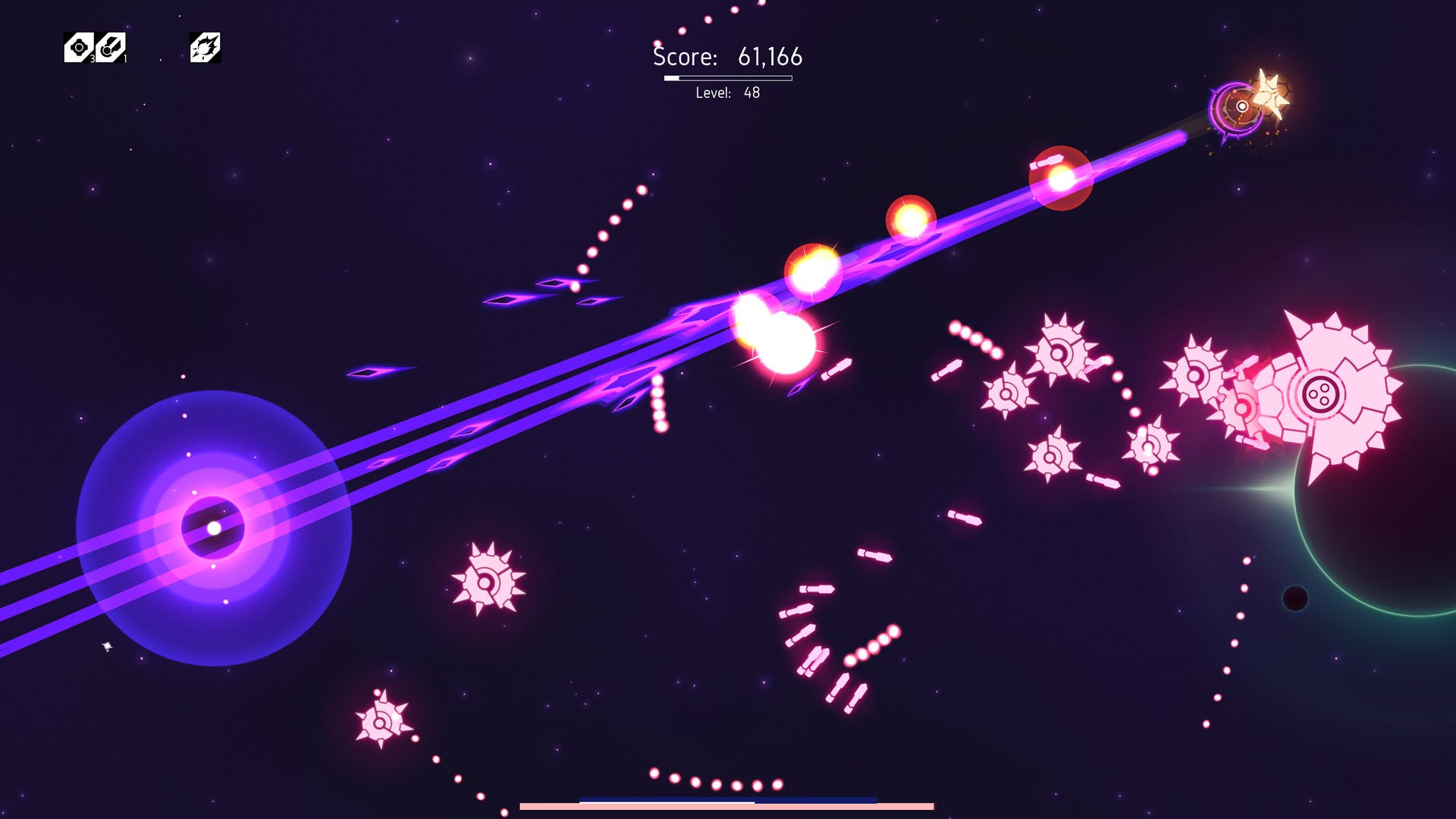
I'm no shoot 'em up nutter - or "shmutter", as I understand they prefer to be called - but some of the first games I remember playing are shmups. Games like Maelstrom, Ambrosia's Macintosh clone of Asteroids, and the proto-shmup Crystal Quest from Patrick Buckland, who would go on to make Carmageddon. Little did I know that the humble premise of a small 2D spacecraft shooting baddies on a wrap-around screen would reach the glittering heights of Nova Drift. Had you shown me this game back in 1995, I dare say I'd have shmupped myself.
MixRift raises $1.6m for mixed reality games
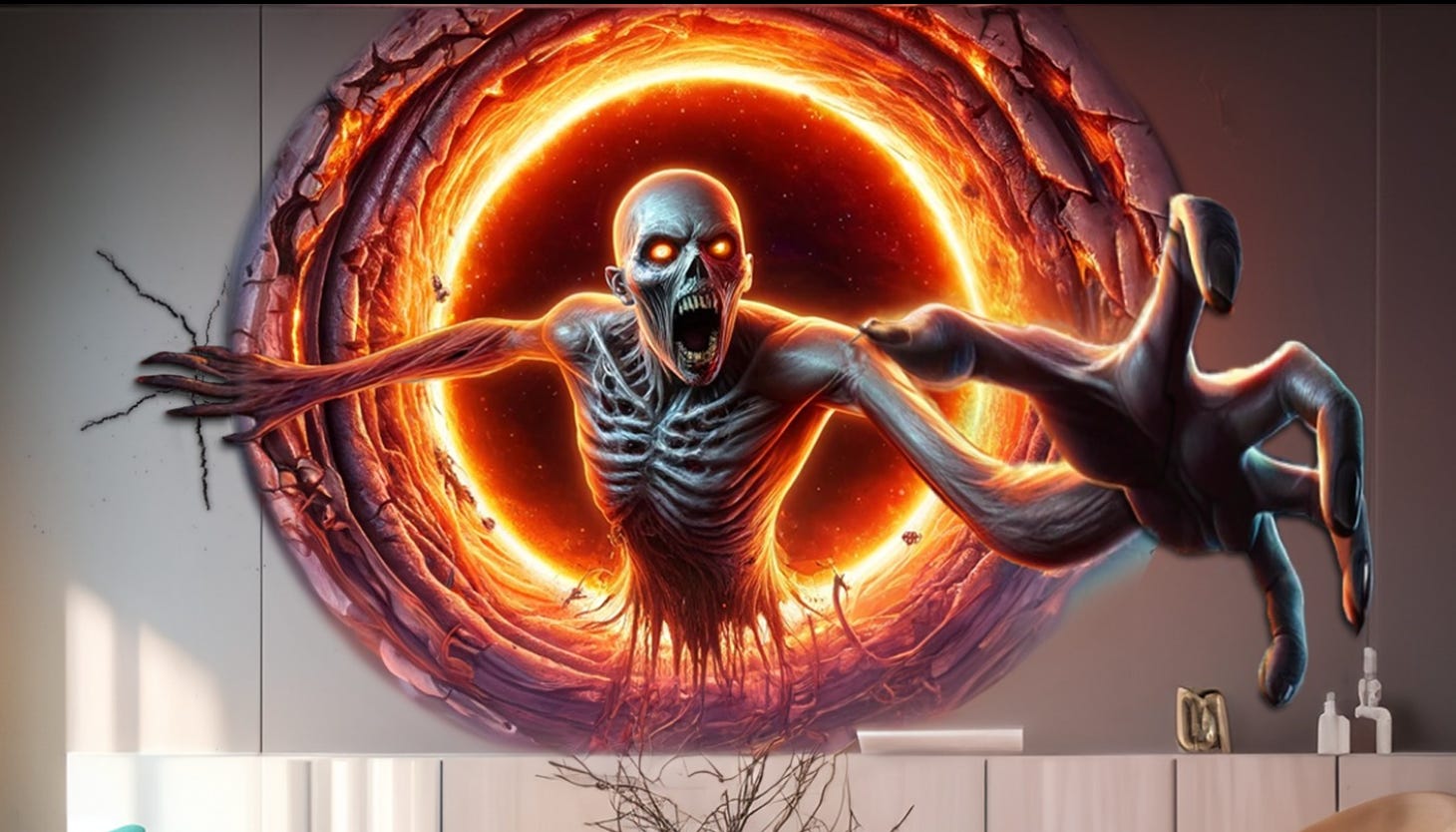
MixRift has raised $1.6 million in a pre-seed funding round.
Outsized Ventures and Underline Ventures co-led the round, with additional contributions from SOSV and angel investors. The startup intends on using the funding to further develop and publish mixed reality titles.
MixRift was co-founded by CEO Bobby Voicu (previously CEO of social games studio MavenHut), CPO David Pripas (formerly creative director at Augmented Studios) and CTO Andrei Vaduva (previously lead engineer at Green Horse Games). All three of them worked together at MavenHut Games in the past.
First Impression: Cave Digger 2 (PC – Steam) ~ No Feedback

One of my favorite activities in Minecraft is going deep inside the caves and just exploring them. A few years ago, the developers behind Cave Digger reached out to me and asked me to review their game. Not too long after, the sequel got released and looked like it would be a VR exclusive. Until I noticed that it appeared on the Nintendo Switch eShop. So, I thought, maybe it also released on Steam, since after playing the Switch version, I felt like this game was better played with keyboard and mouse. Now, a non VR version is on Steam now… But is it worth it? Well, after playing the first sections of this game, I want to talk about it. The latest update was on May 28th, 2024 when writing this article. Now, before we dive right into it, I want to invite to you leave a comment in the comment section with your thoughts and/or opinions on this game and/or the content of this article.
Risk of Staleness

In this game, we play as an unnamed miner who is throwing into the deep end, when his digger broke. You arrive at a mysterious valley. In this valley, a hardy explorer once did his research. But why? Which secrets are in these valleys and the accompanying mines? That’s for our miner to figure out. Now, the story is being told by various comic book pages you can uncover and, according to the Steam store page, has multiple endings. I’m quite curious where it’s going to go.
So far, I haven’t gotten too deep into the story. But, from what I can read on the Steam store page, I think it has potential. I have my doubts on how the multiple endings will work. Since comic books mostly have one ending, right? Unless, it all depends on which page(s) you find or in which order or where. That’s something I’ll discover when I’m deeper into the game.
If this game is like the original game, the story overall will take a backseat for the gameplay. And after 5 hours in, that’s the case. The original game didn’t have a lot of story to begin with, but more story in a game like this can be interesting.
There is one voice actor in this game. He does a pretty fine job and brings some life to the atmosphere. I replayed a bit of the first game and I have to be honest, I appreciate the small voice lines during the exploration. Even when you quickly hear every different line, it’s a nice break since they aren’t spammed and don’t appear that often.
One of the biggest changes in this game is that the cave this time around is randomly generated each time you enter. So, this game becomes a rouge like to a degree. But, you can always exit via the lifts to safety. Since, dying in the caves means that at least half of your obtained loot is dropped. The atmosphere this time around is very cohesive. This game presents itself as a sci-fi western game, and it really feels like that. Something I really like in this game is that it doesn’t go overboard in the sci-fi genre and stays grounded. The technology could realistically exist today, apart from the unique enemies in the cave, that is.
With the story taking more of a backseat, it’s quite important that the gameplay loop is enjoyable. The gameplay loop is simple, you have to explore the caves with 4 chosen tools. The three slots above the entrance give you a hint on which tools you will need to bring to gather the most loot. You take the lift down and gather loot, while fighting enemies and avoiding pitfalls to survive. The goal is also to find the other elevator that takes you down to the next level to gather even more valuable ores to bring to the top. You have to fill in the ores you gathered into the grinder to buy upgrades to your tools and environment to progress.
The big risk with this kind of gameplay loop is that this is just a different numbers game. What I mean by that is that, apart from maybe the visuals changing, the core concept is always the same. This risks that the game becomes stale and repetitive. It’s possible that it is just a “me thing”, but I enjoy games like this more when there are some variations on the gameplay or some different puzzles. Thankfully, this game has that. There are a lot of things you can upgrade and improve to make each run feel rewarding, and each type of cave you can visit has different enemies types and unique lay-outs to keep you on your toes. In a way, I dare to compare the idea a bit to Cult of the Lamb in a degree.
The music in this game is also a blast. It fits the atmosphere of each area like a glove. My favorite track is the track that plays in the lake caves. It sounds like you image a typical track like that to sound. And it gets more intense while you are fighting enemies down there. Now, the silent moments when the music doesn’t play feel a bit long, but I always know that there is more music coming and that it fits the atmosphere perfectly and draws me more into the game. Sadly enough, this isn’t the only problem with this game, and I’d like to talk about them.
No feedback

This game has an addictive gameplay loop, and I’m really curious how the multiplayer works. I haven’t tested the multiplayer in this game, but it looks like fun. Now, this game can be played solo perfectly fine.
Now, I don’t know if VRKiwi took the VR version as a base for the non VR version, since I have the impression, that is the case. I especially notice that with the controls in this game. It feels a bit floaty, like you aren’t really connected to the ground. It also feels a bit stiff, like you have to move your mouse like you would a VR headset. You really have to play with the settings until you hit that sweetspot that feels right for you. For me, I had to lower the sensitivity to 80, amongst other things. I highly recommend that you tweak the settings to your liking, since on the Nintendo Switch version, I had to lower the sensitivity to 40 before it felt right.
Still, the character control doesn’t feel right. At first, I thought it was because the controls felt floaty… But, after some testing, I think I found a few other problems with the character control that might cause it to not feel quite right. First, the jump in this game is just silly. You can’t really rely on it, since it doesn’t always trigger when you hit the spacebar, and it’s just a pathetic jump. You can’t even jump out of ankle high water sometimes.
Secondly, there are no sound effects for walking on most floors. You feel like you are floating, and it’s jarring when you suddenly hear a sound effect when you walk over a table or a railway. Thirdly, climbing on ropes amongst other things is just insanely picky. There is also no real feedback or sound to show you grabbed the rope. Fourthly, the scroll order between tools is extremely weird. You get numbers on the wheel counter clock wise. But you go down, right, left, up. Which still confuses me after 6 hours of playing this game.
And finally, some things are extremely picky. For example, there are safe riddles you can solve down in the caves. But to rotate the letter wheels to make pick the right letter is more difficult to do. All of these things give you a feeling that you aren’t always in control of your character and that you don’t get the feedback as a player on what’s happening. Making you unsure what’s happening and doubt if you are doing the right thing.
Prompts like “Use W/S to use the crank” should be “Hold W/S to use the crank”. Since, you need to hold the key instead of pressing it. Small things like that could also improve this game and it’s controls quite a lot. Overall, the controls are good, but they lack feedback to the player sometimes. Either with sound effects or with some visual effects. Like with the hammer, you barely have any sound effects when you use it, and it has some wind up animation, making you unsure if you are using it or not.
That is one of the biggest flaws in this game. The lack of feedback on your actions. Things like not knowing how many bullets are still left in your revolver or a sound effect when you hit an actual enemy. I think if there is one thing I’d use the built-in feedback tool is to report various cases/moments when I expect feedback from the game, like a sound effect or visual effect. Maybe they appear in the form of rumble effects… But, I’m not playing this game with a controller.
When you read this section of the article, I wouldn’t blame you if you think that this game isn’t good. Small bugs like the text of “Press R to reload” when your gun isn’t equipped or the bullets not leaving from the gun but from the player model don’t improve things either. Yet, I find myself looking past these problems since the core gameplay still works. I find myself getting used to the jank in this game and finding a very rough diamond. If the developers keep up with their promise of improving this game, I think that more action feedback will bring a lot to the game and maybe fixing the small bugs like in this paragraph as well.
Things like the animation of the shovel looking weird sometimes. The animation looks like the arms go through each other after a dig. Speaking of the shovel, the last dig is annoying since you have to move a pixel or two for it to count and give you your goodies. But the bug I’d love to see fixed most is the freeze for several seconds when you pick up something new or get a new codec entry. The game locks up like it’s about the crash, but it doesn’t.
What’s next for us?

Usually, I’m not really picky when it comes to the visuals of a game. As long as a game looks consistent, I’m quite happy. It needs to have a certain style so that you can quickly identify what’s what and enjoy the game.
Yet, for this game, I do have some things that I not really like in terms of the visuals. Firstly, the contrast of some ores and the floor isn’t clear enough. Sometimes I was passing up on ores since I wasn’t able to notice them on the ground.
There are also a lot of objects to give more details to the cave, but you can barely interact with them. I’d love to see lilly pads in lakes to move a bit when you walk past them or something more than just being able to clip through them. As well, a sound effect when you hit a wall you can’t mine. You get shouted at when you use the wrong or a too weak tool on something, so when not for the rest?
I think the biggest mistake that the visuals make is that it has an identity crisis. What I mean by that is that it isn’t a cohesive style. There is a lot of shell shading going on, but there is also a lot of details that give off a more realistic vibe. Some textures aren’t detailed enough and strechted too wide giving wrong impression the rest of the visuals that look more modern. The floor textures sometimes suffer most from this issue.
Looking back at this article, I think I’m being very critical for this game. I have played a lot worse and broken games for 15€. But, in this game you even have customisation options for your character and thee developers are extremely open for feedback. This game has a lot going for it. Fun achievements to hunt for, bosses at the end of runs and an amazing auto save system.
Apart from improving the character controls and adding some feedback on actions, I think this game is pretty decent. Yes, there is some polish missing like not having a tooltip with the lever at the cave entrance on what that lever does. I personally feel less conflicted about this game compared to the original. The growth in this title is immense and brings me a lot of hope for either some amazing updates, DLC or a new entry in the series.
The basis of for an amazing title is here and if you look past it’s short comings, this game is a blast to play. Maybe it’s a bit too repetitive for some and can be more fun in short bursts. But, when this game sinks it’s hooks into you, it really clicks. There is some polishing left to do and for a rather new VR focused developer, this is amazing. It’s their second non VR game and it shows a lot of promise.
The game is a perfect relaxing game to wind down, since it isn’t too difficult. The game is rather forgiving. I wouldn’t be surprised that I play this game after work to wind down and try and finish it slowly. Then again, while I’m writing this, I have summer holidays and I wouldn’t be surprised that I finish most of this game during my summer break.
Like I said earlier, I feel less conflicted about this game compared to the previous title. This game has a lot more going for it compared to the original. It’s less repetitive and it has a lot more going for it. It has it’s problems, yes. But, if you enjoy games like Minecraft, Steamworld Dig or Cave Digger, give the demo of this game a chance. The demo gives a very good idea on what you can expect from this game and if you enjoy it, buy the game. I’m enjoying myself quite a lot with this game and I’m happy that I have chosen the PC version over the Switch version since I feel like it just plays better. But maybe, if I get used to the Switch controls, I might enjoy it on Switch as well.
With that said, I have said everything I wanted to say about this game for now. Maybe when I finish this game, I might write a full review with the final thoughts and opinions on this game. But for now, I think the best conclusion for this game is that it’s an amazing step up from the original and besides some unpolished things… It’s a great game and comes recommend from me.
So, it’s time to wrap up this article with my usual outro. I hope you enjoyed reading it as much as I enjoyed writing it. I hope to be able to welcome you in another article, but until then have a great rest of your day and take care.
Makoto Wakaido’s Case Files Trilogy Deluxe Review – Pixel Art Detective Mysteries
Wisdom of Nym: The story beats that really work in Final Fantasy XIV Dawntrail
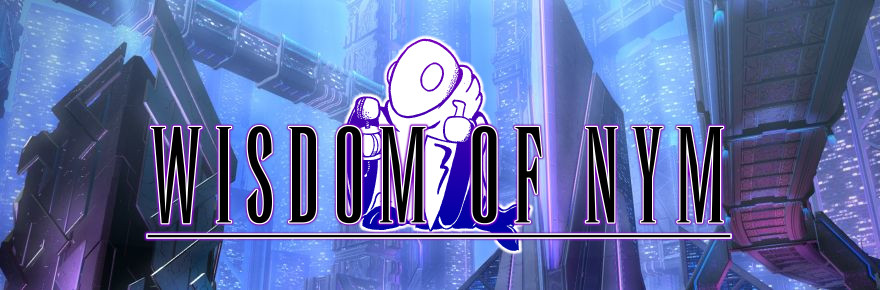
Embers Adrift promises a ‘crazy’ weekend of in-game events starting August 16
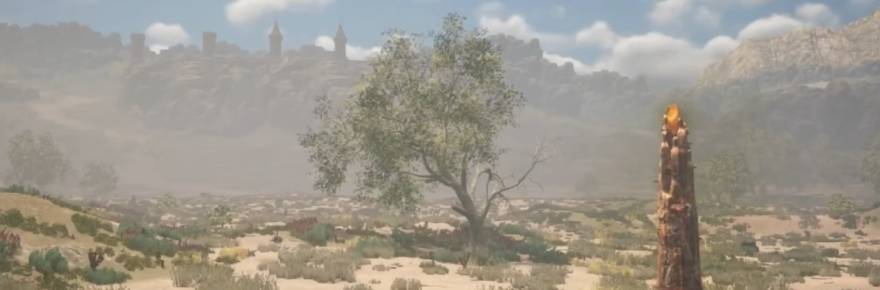
Makoto Wakaido’s Case Files Trilogy Deluxe Review – Pixel Art Detective Mysteries
No Man’s Sky Just Became A Desolate Space Horror Game
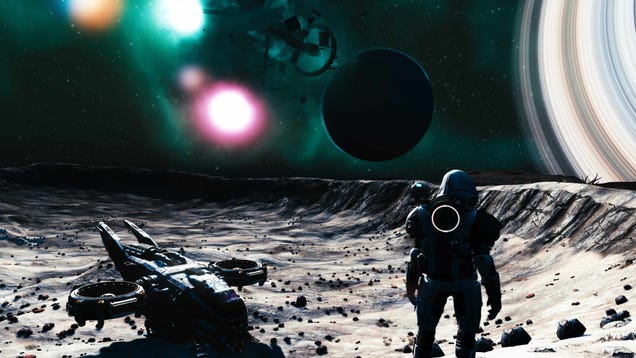
It’s been about eight years since No Man’s Sky first launched and it continues to get updates that radically transform the game. Its latest update, Adrift, remains ambitious as it drops players into what Hello Games is calling “an abandoned universe” with no shops and no NPCs. You are the only intelligent life in the…
Predicting And Preventing Process Drift
Increasingly tight tolerances and rigorous demands for quality are forcing chipmakers and equipment manufacturers to ferret out minor process variances, which can create significant anomalies in device behavior and render a device non-functional.
In the past, many of these variances were ignored. But for a growing number of applications, that’s no longer possible. Even minor fluctuations in deposition rates during a chemical vapor deposition (CVD) process, for example, can lead to inconsistencies in layer uniformity, which can impact the electrical isolation properties essential for reliable circuit operation. Similarly, slight variations in a photolithography step can cause alignment issues between layers, leading to shorts or open circuits in the final device.
Some of these variances can be attributed to process error, but more frequently they stem from process drift — the gradual deviation of process parameters from their set points. Drift can occur in any of the hundreds of process steps involved in manufacturing a single wafer, subtly altering the electrical properties of chips and leading to functional and reliability issues. In highly complex and sensitive ICs, even the slightest deviations can cause defects in the end product.
“All fabs already know drift. They understand drift. They would just like a better way to deal with drift,” said David Park, vice president of marketing at Tignis. “It doesn’t matter whether it’s lithography, CMP (chemical mechanical polishing), CVD or PVD (chemical/physical vapor deposition), they’re all going to have drift. And it’s all going to happen at various rates because they are different process steps.”
At advanced nodes and in dense advanced packages, where a nanometer can be critical, controlling process drift is vital for maintaining high yield and ensuring profitability. By rigorously monitoring and correcting for drift, engineers can ensure that production consistently meets quality standards, thereby maximizing yield and minimizing waste.
“Monitoring and controlling hundreds of thousands of sensors in a typical fab requires the ability to handle petabytes of real-time data from a large variety of tools,” said Vivek Jain, principal product manager, smart manufacturing at Synopsys. “Fabs can only control parameters or behaviors they can measure and analyze. They use statistical analysis and error budget breakdowns to define upper control limits (UCLs) and lower control limits (LCLs) to monitor the stability of measured process parameters and behaviors.”
Dialing in legacy fabs
In legacy fabs — primarily 200mm — most of the chips use 180nm or older process technology, so process drift does not need to be as precisely monitored as in the more advanced 300mm counterparts. Nonetheless, significant divergence can lead to disparities in device performance and reliability, creating a cascade of operational challenges.
Manufacturers operating at older technology nodes might lack the sophisticated, real-time monitoring and control methods that are standard in cutting-edge fabs. While the latter have embraced ML to predict and correct for drift, many legacy operations still rely heavily on periodic manual checks and adjustments. Thus, the management of process drift in these settings is reactive rather than proactive, making changes after problems are detected rather than preventing them.
“There is a separation between 300-millimeter and 200-millimeter fabs,” said Park. “The 300-millimeter guys are all doing some version of machine learning. Sometimes it’s called advanced process control, and sometimes it’s actually AI-powered process control. For some of the 200-millimeter fabs with more mature process nodes, they basically have a recipe they set and a bunch of technicians looking at machines and looking at the CDs. When the drift happens, they go through their process recipe and manually adjust for the out-of-control processes, and that’s just what they’ve always done. It works for them.”
For these older fabs, however, the repercussions of process drift can be substantial. Minor deviations in process parameters, such as temperature or pressure during the deposition or etching phases, gradually can lead to changes in the physical structure of the semiconductor devices. Over time, these minute alterations can compound, resulting in layers of materials that deviate from their intended characteristics. Such deviations affect critical dimensions and ultimately can compromise the electrical performance of the chip, leading to slower processing speeds, higher power consumption, or outright device failure.
The reliability equation is equally impacted by process drift. Chips are expected to operate consistently over extended periods, often under a range of environmental conditions. However, when process-induced variability can weaken the device’s resilience, precipitating early wear-out mechanisms and reducing its lifetime. In situations where dependability is non-negotiable, such as in automotive or medical applications, those variations can have dire consequences.
But with hundreds of process steps for a typical IC, eliminating all variability in fabs is simply not feasible.
“Process drift is never going to not happen, because the processes are going to have some sort of side effect,” Park said. “The machines go out of spec and things like pumps and valves and all sorts of things need to be replaced. You’re still going to have preventive maintenance (PM). But if the critical dimensions are being managed correctly, which is typically what triggers the drift, you can go a longer period of time between cleanings or the scheduled PMs and get more capacity.”
Process drift pitfalls
Managing process drift in semiconductor manufacturing presents several complex challenges. Hysteresis, for example, is a phenomenon where the output of a process varies not solely because of current input conditions, but also based on the history of the states through which the process already has passed. This memory effect can significantly complicate precision control, as materials and equipment might not reset to a baseline state after each operational cycle. Consequently, adjustments that were effective in previous cycles may not yield the same outcomes due to accumulated discrepancies.
One common cause of hysteresis is thermal cycling, where repeated heating and cooling create mechanical stresses. Those stresses can be additive, releasing inconsistently based on temperature history. That, in turn, can lead to permanent changes in the output of a circuit, such as a voltage reference, which affects its precision and stability.
In many field-effect transistors (FETs), hysteresis also can occur due to charge trapping. This happens when charges are captured in ‘trap states’ within the semiconductor material or at the interface with another material, such as an oxide layer. The trapped charges then can modulate the threshold voltage of the device over time and under different electrical biases, potentially leading to operational instability and variability in device performance.
Human factors also play a critical role in process drift, with errors stemming from incorrect settings adjustments, mishandling of materials, misinterpretation of operational data, or delayed responses to process anomalies. Such errors, though often minor, can lead to substantial variations in manufacturing processes, impacting the consistency and reliability of semiconductor devices.
“Once in production, the biggest source of variability is human error or inconsistency during maintenance,” said Russell Dover, general manager of service product line at Lam Research. “Wet clean optimization (WCO) and machine learning through equipment intelligence solutions can help address this.”
The integration of new equipment into existing production lines introduces additional complexities. New machinery often features increased speed, throughput, and tighter tolerances, but it must be integrated thoughtfully to maintain the stringent specifications required by existing product lines. This is primarily because the specifications and performance metrics of legacy chips have been long established and are deeply integrated into various applications with pre-existing datasheets.
“From an equipment supplier perspective, we focus on tool matching,” said Dover. “That includes manufacturing and installing tools to be identical within specification, ensuring they are set up and running identically — and then bringing to bear systems, tooling, software and domain knowledge to ensure they are maintained and remain as identical as possible.”
The inherent variability of new equipment, even those with advanced capabilities, requires careful calibration and standardization.
“Some equipment, like transmission electron microscopes, are incredibly powerful,” said Jian-Min Zuo, a materials science and engineering professor at the University of Illinois’ Grainger College of Engineering. “But they are also very finicky, depending on how you tune the machine. How you set it up under specific conditions may vary slightly every time. So there are a number of things that can be done when you try to standardize those procedures, and also standardize the equipment. One example is to generate a curate, like a certain type of test case, where you can collect data from different settings and make sure you’re taking into account the variability in the instruments.”
Process drift solutions
As semiconductor manufacturers grapple with the complexities of process drift, a diverse array of strategies and tools has emerged to address the problem. Advanced process control (APC) systems equipped with real-time monitoring capabilities can extract patterns and predictive insights from massive data sets gathered from various sensors throughout the manufacturing process.
By understanding the relationships between different process variables, APC can predict potential deviations before they result in defects. This predictive capability enables the system to make autonomous adjustments to process parameters in real-time, ensuring that each process step remains within the defined control limits. Essentially, APC acts as a dynamic feedback mechanism that continuously fine-tunes the production process.
Fig. 1: Reduced process drift with AI/ML advanced process control. Source: Tignis
While APC proactively manages and optimizes the process to prevent deviations, fault detection and classification (FDC) reacts to deviations by detecting and classifying any faults that still occur.
FDC data serves as an advanced early-warning system. This system monitors the myriad parameters and signals during the chip fabrication process, rapidly detecting any variances that could indicate a malfunction or defect in the production line. The classification component of FDC is particularly crucial, as it does more than just flag potential issues. It categorizes each detected fault based on its characteristics and probable causes, vastly simplifying the trouble-shooting process. This allows engineers to swiftly pinpoint the type of intervention needed, whether it’s recalibrating instruments, altering processing recipes, or conducting maintenance repairs.
Statistical process control (SPC) is primarily focused on monitoring and controlling process variations using statistical methods to ensure the process operates efficiently and produces output that meets quality standards. SPC involves plotting data in real-time against control limits on control charts, which are statistically determined to represent the expected normal process behavior. When process measurements stray outside these control limits, it signals that the process may be out of control due to special causes of variation, requiring investigation and correction. SPC is inherently proactive and preventive, aiming to detect potential problems before they result in product defects.
“Statistical process control (SPC) has been a fundamental methodology for the semiconductor industry almost from its very foundation, as there are two core factors supporting the need,” said Dover. “The first is the need for consistent quality, meaning every product needs to be as near identical as possible, and second, the very high manufacturing volume of chips produced creates an excellent workspace for statistical techniques.”
While SPC, FDC, and APC might seem to serve different purposes, they are deeply interconnected. SPC provides the baseline by monitoring process stability and quality over time, setting the stage for effective process control. FDC complements SPC by providing the tools to quickly detect and address anomalies and faults that occur despite the preventive measures put in place by SPC. APC takes insights from both SPC and FDC to adjust process parameters proactively, not just to correct deviations but also to optimize process performance continually.
Despite their benefits, integrating SPC, FDC and APC systems into existing semiconductor manufacturing environments can pose challenges. These systems require extensive configuration and tuning to adapt to specific manufacturing conditions and to interface effectively with other process control systems. Additionally, the success of these systems depends on the quality and granularity of the data they receive, necessitating high-fidelity sensors and a robust data management infrastructure.
“For SPC to be effective you need tight control limits,” adds Dover. “A common trap in the world of SPC is to keep adding control charts (by adding new signals or statistics) during a process ramp, or maybe inheriting old practices from prior nodes without validating their relevance. The result can be millions of control charts running in parallel. It is not a stretch to state that if you are managing a million control charts you are not really controlling much, as it is humanly impossible to synthesize and react to a million control charts on a daily basis.”
This is where AI/ML becomes invaluable, because it can monitor the performance and sustainability of the new equipment more efficiently than traditional methods. By analyzing data from the new machinery, AI/ML can confirm observations, such as reduced accumulation, allowing for adjustments to preventive maintenance schedules that differ from older equipment. This capability not only helps in maintaining the new equipment more effectively but also in optimizing the manufacturing process to take full advantage of the technological upgrades.
AI/ML also facilitate a smoother transition when integrating new equipment, particularly in scenarios involving ‘copy exact’ processes where the goal is to replicate production conditions across different equipment setups. AI and ML can analyze the specific outputs and performance variations of the new equipment compared to the established systems, reducing the time and effort required to achieve optimal settings while ensuring that the new machinery enhances production without compromising the quality and reliability of the legacy chips being produced.
AI/ML
Being more proactive in identifying drift and adjusting parameters in real-time is a necessity. With a very accurate model of the process, you can tune your recipe to minimize that variability and improve both quality and yield.
“The ability to quickly visualize a month’s worth of data in seconds, and be able to look at windows of time, is a huge cost savings because it’s a lot more involved to get data for the technicians or their process engineers to try and figure out what’s wrong,” said Park. “AI/ML has a twofold effect, where you have fewer false alarms, and just fewer alarms in general. So you’re not wasting time looking at things that you shouldn’t have to look at in the first place. But when you do find issues, AI/ML can help you get to the root cause in the diagnostics associated with that much more quickly.”
When there is a real alert, AI/ML offers the ability to correlate multiple parameters and inputs that are driving that alert.
“Traditional process control systems monitor each parameter separately or perform multivariate analysis for key parameters that require significant effort from fab engineers,” adds Jain. “With the amount of fab data scaling exponentially, it is becoming humanly impossible to extract all the actionable insights from the data. Machine learning and artificial intelligence can handle big data generated within a fab to provide effective process control with minimal oversight.”
AI/ML also can look for more other ways of predicting when the drift is going to take your process out of specification. Those correlations can be bivariate and multivariate, as well as univariate. And a machine learning engine that is able to sift through tremendous amounts of data and a larger number of variables than most humans also can turn up some interesting correlations.
“Another benefit of AI/ML is troubleshooting when something does trigger an alarm or alert,” adds Park. “You’ve got SPC and FDC that people are using, and a lot of them have false positives, or false alerts. In some cases, it’s as high as 40% of the alerts that you get are not relevant for what you’re doing. This is where AI/ML becomes vital. It’s never going to take false alerts to zero, but it can significantly reduce the amount of false alerts that you have.”
Engaging with these modern drift solutions, such as AI/ML-based systems, is not mere adherence to industry trends but an essential step towards sustainable semiconductor production. Going beyond the mere mitigation of process drift, these technologies empower manufacturers to optimize operations and maintain the consistency of critical dimensions, allowed by the intelligent analysis of extensive data and automation of complex control processes.
Conclusion
Monitoring process drift is essential for maintaining quality of the device being manufactured, but it also can ensure that the entire fabrication lifecycle operates at peak efficiency. Detecting and managing process drift is a significant challenge in volume production because these variables can be subtle and may compound over time. This makes identifying the root cause of any drift difficult, particularly when measurements are only taken at the end of the production process.
Combating these challenges requires a vigilant approach to process control, regular equipment servicing, and the implementation of AI/ML algorithms that can assist in predicting and correcting for drift. In addition, fostering a culture of continuous improvement and technological adaptation is crucial. Manufacturers must embrace a mindset that prioritizes not only reactive measures, but also proactive strategies to anticipate and mitigate process drift before it affects the production line. This includes training personnel to handle new technologies effectively and to understand the dynamics of process control deeply. Such education enables staff to better recognize early signs of drift and respond swiftly and accurately.
Moreover, the integration of comprehensive data analytics platforms can revolutionize how fabs monitor and analyze the vast amounts of data they generate. These platforms can aggregate data from multiple sources, providing a holistic view of the manufacturing process that is not possible with isolated measurements. With these insights, engineers can refine their process models, enhance predictive maintenance schedules, and optimize the entire production flow to reduce waste and improve yields.
Related Reading
Tackling Variability With AI-Based Process Control
How AI in advanced process control reduces equipment variability and corrects for process drift.
The post Predicting And Preventing Process Drift appeared first on Semiconductor Engineering.
Tackling Variability With AI-based Process Control
Jon Herlocker, co-founder and CEO of Tignis, sat down with Semiconductor Engineering to talk about how AI in advanced process control reduces equipment variability and corrects for process drift. What follows are excerpts of that conversation.
SE: How is AI being used in semiconductor manufacturing and what will the impact be?
Herlocker: AI is going to create a completely different factory. The real change is going to happen when AI gets integrated, from the design side all the way through the manufacturing side. We are just starting to see the beginnings of this integration right now. One of the biggest challenges in the semiconductor industry is it can take years from the time an engineer designs a new device to that device reaching high-volume production. Machine learning is going to cut that to half, or even a quarter. The AI technology that Tignis offers today accelerates that very last step — high-volume manufacturing. Our customers want to know how to tune their tools so that every time they process a wafer the process is in control. Traditionally, device makers get the hardware that meets their specifications from the equipment manufacturer, and then the fab team gets their process recipes working. Depending on the size of the fab, they try to physically replicate that process in a ‘copy exact’ manner, which can take a lot of time and effort. But now device makers can use machine learning (ML) models to autonomously compensate for the differences in equipment variation to produce the exact same outcome, but with significantly less effort by process engineers and equipment technicians.
SE: How is this typically done?
Herlocker: A classic APC system on the floor today might model three input parameters using linear models. But if you need to model 20 or 30 parameters, these linear models don’t work very well. With AI controllers and non-linear models, customers can ingest all of their rich sensor data that shows what is happening in the chamber, and optimally modulate the recipe settings to ensure that the outcome is on-target. AI tools such as our PAICe Maker solution can control any complex process with a greater degree of precision.
SE: So, the adjustments AI process control software makes is to tweak inputs to provide consistent outputs?
Herlocker: Yes, I preach this all the time. By letting AI automate the tasks that were traditionally very manual and time-consuming, engineers and technicians in the fab can remove a lot of the manual precision tasks they needed to do to control their equipment, significantly reducing module operating costs. AI algorithms also can help identify integration issues — interacting effects between tools that are causing variability. We look at process control from two angles. Software can autonomously control the tool by modulating the recipe parameters in response to sensor readings and metrology. But your autonomous control cannot control the process if your equipment is not doing what it is supposed to do, so we developed a separate AI learning platform that ensures equipment is performing to specification. It brings together all the different data silos across the fab – the FDC trace data, metrology data, test data, equipment data, and maintenance data. The aggregation of all that data is critical to understanding the causes of a variation in equipment. This is where ML algorithms can automatically sift through massive amount of data to help process engineers and data scientists determine what parameters are most influencing their process outcomes.
SE: Which process tools benefit the most from AI modeling of advanced process control?
Herlocker: We see the most interest in thin film deposition tools. The physics involved in plasma etching and plasma-enhanced CVD are non-linear processes. That is why you can get much better control with ML modeling. You also can model how the process and equipment evolves over time. For example, every time you run a batch through the PECVD chamber you get some amount of material accumulation on the chamber walls, and that changes the physics and chemistry of the process. AI can build a predictive model of that chamber. In addition to reacting to what it sees in the chamber, it also can predict what the chamber is going to look like for the next run, and now the ML model can tweak the input parameters before you even see the feedback.
SE: How do engineers react to the idea that the AI will be shifting the tool recipe?
Herlocker: That is a good question. Depending on the customer, they have different levels of comfort about how frequently things should change, and how much human oversight there needs to be for that change. We have seen everything from, ‘Just make a recommendation and one of our engineers will decide whether or not to accept that recommendation,’ to adjusting the recipe once a day, to autonomously adjusting for every run. The whole idea behind these adjustments is for variability reduction and drift management, and customers weigh the targeted results versus the perceived risk of taking a novel approach.
SE: Does this involve building confidence in AI-based approaches?
Herlocker: Absolutely, and our systems have a large number of fail-safes, and some limits are hard-coded. We have people with PhDs in chemical engineering and material science who have operated these tools for years. These experts understand the physics of what is happening in these tools, and they have the practical experience to know what level of change can be expected or not.
SE: How much of your modeling is physics-based?
Herlocker: In the beginning, all of our modeling was physics-based, because we were working with equipment makers on their next-generation tools. But now we are also bringing our technology to device makers, where we can also deliver a lot of value by squeezing the most juice out of a data-driven approach. The main challenge with physics models is they are usually IP-protected. When we work with equipment makers, they typically pay us to build those physics-based models so they cannot be shared with other customers.
SE: So are your target customers the toolmakers or the fabs?
Herlocker: They are both our target customers. Most of our sales and marketing efforts are focused on device makers with legacy fabs. In most cases, the fab manager has us engage with their team members to do an assessment. Frequently, that team includes a cross section of automation, process, and equipment teams. The automation team is most interested in reducing the time to detect some sort of deviation that is going to cause yield loss, scrap, or tool downtime. The process and equipment engineers are interested in reducing variability or controlling drift, which also increases chamber life.
For example, let’s consider a PECVD tool. As I mentioned, every time you run the process, byproducts such as polymer materials build up on the chamber walls. You want a thickness of x in your deposition, but you are getting a slightly different wafer thickness uniformity due to drift of that chamber because of plasma confinement changes. Eventually, you must shut down the tool, wet clean the chamber, replace the preventive maintenance kit parts, and send them through the cleaning loop (i.e., to the cleaning vendor shop). Then you need to season the chamber and bring it back online. By controlling the process better, the PECVD team does not have to vent the chamber as often to clean parts. Just a 5% increase in chamber life can be quite significant from a maintenance cost reduction perspective (e.g., parts spend, refurb spend, cleaning spend, etc.). Reducing variability has a similarly large impact, particularly if it is a bottleneck tool, because then that reduction directly contributes to higher or more stable yields via more ‘sweet spot’ processing time, and sometimes better wafer throughput due to the longer chamber lifetime. The ROI story is more nuanced on non-bottleneck tools because they don’t modulate fab revenue, but the ROI there is still there. It is just more about chamber life stability.
SE: Where does this go next?
Herlocker: We also are working with OEMs on next-generation toolsets. Using AI/ML as the core of process control enables equipment makers to control processes that are impossible to implement with existing control strategies and software. For example, imagine on each process step there are a million different parameters that you can control. Further imagine that changing any one parameter has a global effect on all the other parameters, and only by co-varying all the million parameters in just the right way will you get the ideal outcome. And to further complicate things, toss in run-to-run variance, so that the right solution continues to change over time. And then there is the need to do this more than 200 times per hour to support high-volume manufacturing. AI/ML enables this kind of process control, which in turn will enable a step function increase in the ability to produce more complex devices more reliably.
SE: What additional changes do you see from AI-based algorithms?
Herlocker: Machine learning will dramatically improve the agility and productivity of the facility broadly. For example, process engineers will spend less time chasing issues and have more time to implement continuous improvement. Maintenance engineers will have time to do more preventive maintenance. Agility and resiliency — the ability to rapidly adjust to or maintain operations, despite disturbances in the factory or market — will increase. If you look at ML combined with upcoming generative AI capabilities, within a year or two we are going to have agents that effectively will understand many aspects of how equipment or a process works. These agents will make good engineers great, and enable better capture, aggregation, and transfer of manufacturing knowledge. In fact, we have some early examples of this running in our labs. These ML agents capture and ingest knowledge very quickly. So when it comes to implementing the vision of smart factories, machine learning automation will have a massive impact on manufacturing in the future.
The post Tackling Variability With AI-based Process Control appeared first on Semiconductor Engineering.