Empowering undergraduate computer science students to shape generative AI research
As use of generative artificial intelligence (or generative AI) tools such as ChatGPT, GitHub Copilot, or Gemini becomes more widespread, educators are thinking carefully about the place of these tools in their classrooms. For undergraduate education, there are concerns about the role of generative AI tools in supporting teaching and assessment practices. For undergraduate computer science (CS) students, generative AI also has implications for their future career trajectories, as it is likely to be relevant across many fields.
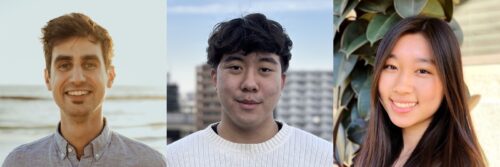
In a recent seminar in our current series on teaching programming (with or without AI), we were delighted to be joined by Dr Stephen MacNeil, Andrew Tran, and Irene Hou from Temple University. Their talk showcased several research projects involving generative AI in undergraduate education, and explored how undergraduate research projects can create agency for students in navigating the implications of generative AI in their professional lives.
Differing perceptions of generative AI
Stephen began by discussing the media coverage around generative AI. He highlighted the binary distinction between media representations of generative AI as signalling the end of higher education — including programming in CS courses — and other representations that highlight the issues that using generative AI will solve for educators, such as improving access to high-quality help (specifically, virtual assistance) or personalised learning experiences.
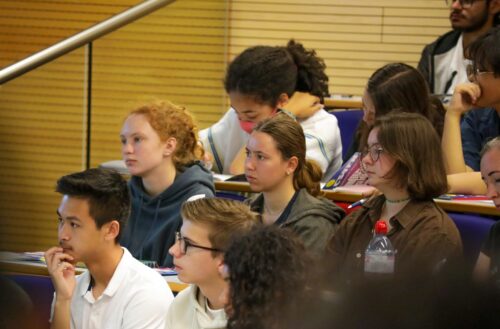
As part of a recent ITiCSE working group, Stephen and colleagues conducted a survey of undergraduate CS students and educators and found conflicting views about the perceived benefits and drawbacks of generative AI in computing education. Despite this divide, most CS educators reported that they were planning to incorporate generative AI tools into their courses. Conflicting views were also noted between students and educators on what is allowed in terms of generative AI tools and whether their universities had clear policies around their use.
The role of generative AI tools in students’ help-seeking
There is growing interest in how undergraduate CS students are using generative AI tools. Irene presented a study in which her team explored the effect of generative AI on undergraduate CS students’ help-seeking preferences. Help-seeking can be understood as any actions or strategies undertaken by students to receive assistance when encountering problems. Help-seeking is an important part of the learning process, as it requires metacognitive awareness to understand that a problem exists that requires external help. Previous research has indicated that instructors, teaching assistants, student peers, and online resources (such as YouTube and Stack Overflow) can assist CS students. However, as generative AI tools are now widely available to assist in some tasks (such as debugging code), Irene and her team wanted to understand which resources students valued most, and which factors influenced their preferences. Their study consisted of a survey of 47 students, and follow-up interviews with 8 additional students.
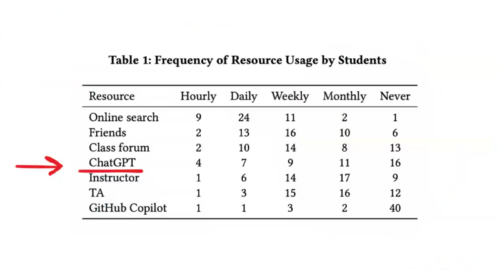
Responding to the survey, students stated that they used online searches or support from friends/peers more frequently than two generative AI tools, ChatGPT and GitHub Copilot; however, Irene indicated that as data collection took place at the beginning of summer 2023, it is possible that students were not familiar with these tools or had not used them yet. In terms of students’ experiences in seeking help, students found online searches and ChatGPT were faster and more convenient, though they felt these resources led to less trustworthy or lower-quality support than seeking help from instructors or teaching assistants.
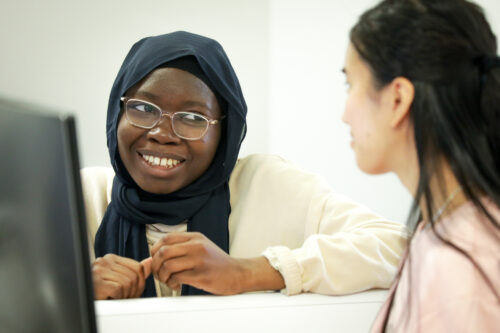
Some students felt more comfortable seeking help from ChatGPT than peers as there were fewer social pressures. Comparing generative AI tools and online searches, one student highlighted that unlike Stack Overflow, solutions generated using ChatGPT and GitHub Copilot could not be verified by experts or other users. Students who received the most value from using ChatGPT in seeking help either (i) prompted the model effectively when requesting help or (ii) viewed ChatGPT as a search engine or comprehensive resource that could point them in the right direction. Irene cautioned that some students struggled to use generative AI tools effectively as they had limited understanding of how to write effective prompts.
Using generative AI tools to produce code explanations
Andrew presented a study where the usefulness of different types of code explanations generated by a large language model was evaluated by students in a web software development course. Based on Likert scale data, they found that line-by-line explanations were less useful for students than high-level summary or concept explanations, but that line-by-line explanations were most popular. They also found that explanations were less useful when students already knew what the code did. Andrew and his team then qualitatively analysed code explanations that had been given a low rating and found they were overly detailed (i.e. focusing on superfluous elements of the code), the explanation given was the wrong type, or the explanation mixed code with explanatory text. Despite the flaws of some explanations, they concluded that students found explanations relevant and useful to their learning.
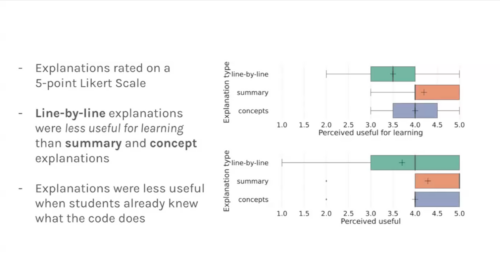
Using generative AI tools to create multiple choice questions
In a separate study, Andrew and his team investigated the use of ChatGPT to generate novel multiple choice questions for computing courses. The researchers prompted two models, GPT-3 and GPT-4, with example question stems to generate correct answers and distractors (incorrect but plausible choices). Across two data sets of example questions, GPT-4 significantly outperformed GPT-3 in generating the correct answer (75.3% and 90% vs 30.8% and 36.7% of all cases). GPT-3 performed less well at providing the correct answer when faced with negatively worded questions. Both models generated correct answers as distractors across both sets of example questions (GPT-3: 11.1% and 10% of cases; GPT-4: 9.9% and 17.8%). They concluded that educators would still need to verify whether answers were correct and distractors were appropriate.
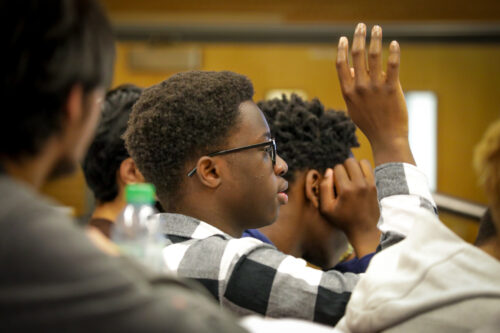
Undergraduate students shaping the direction of generative AI research
With student concerns about generative AI and its implications for the world of work, the seminar ended with a hopeful message highlighting undergraduate students being proactive in conducting their own research and shaping the direction of generative AI research in computer science education. Stephen concluded the seminar by celebrating the undergraduate students who are undertaking these research projects.
You can watch the seminar here:
If you are interested to learn more about Stephen’s work on generative AI, you can read about how undergraduate students used generative AI tools to create analogies for recursion. If you would like to experiment with using generative AI tools to assist with debugging, you could try using Gemini, ChatGPT, or Copilot.
Join our next seminar
Our current seminar series is on teaching programming with or without AI.
In our next seminar, on 16 July at 17:00 to 18:30 BST, we welcome Laurie Gale (Raspberry Pi Computing Education Research Centre, University of Cambridge), who will discuss how to teach debugging to secondary school students. To take part in the seminar, click the button below to sign up, and we will send you information about how to join. We hope to see you there.
The schedule of our upcoming seminars is available online. You can catch up on past seminars on our blog and on the previous seminars and recordings page.
The post Empowering undergraduate computer science students to shape generative AI research appeared first on Raspberry Pi Foundation.